Demystifying AI in Insurance Underwriting and Risk Assessment
According to the McKinsey Report, “By 2030, the insurance underwriting process will be completed in just a few seconds, driven by AI automation.
The insurance industry is undergoing a technological revolution fuelled by the disruptive influence of AI. Traditional risk assessment, underwriting, and claim processing are often time-consuming and labor-intensive, underpinning the necessity for adopting automation and data-driven solutions powered by AI. In the dynamic world of insurance, AI has emerged as a game changer, fundamentally reshaping risk assessments and streamlining underwriting workflows, optimizing pricing strategies to achieve greater accuracy, efficiency, and enhanced customer satisfaction.
In this blog, lets understand how AI can be used in some of the core insurance workflows ultimately benefiting both insurers and policyholders.
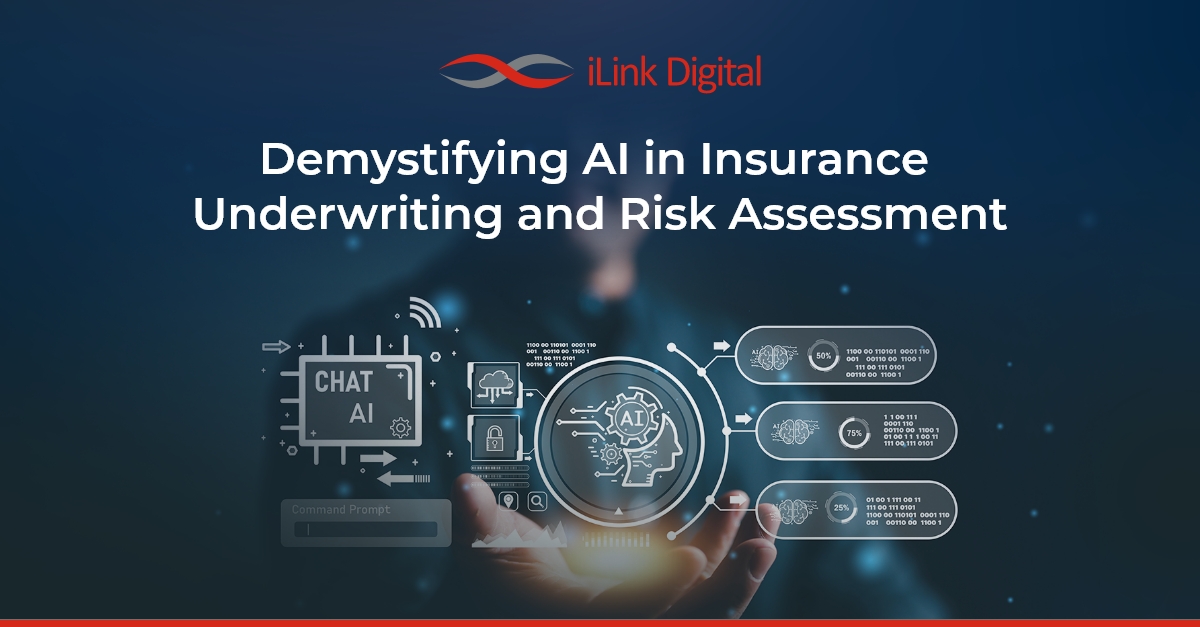
Challenges in Underwriting
Insurance underwriters heavily rely on the total population data and analyse vast amounts of customer data to assess the underlying risk and determine appropriate insurance premiums. Different underwriters may interpret the same information differently, leading to inconsistent and inaccurate risk assessment, that can expose the insurance company to huge claims requests when any such risks occur.
Let’s look at a few challenges that insurance companies face in determining their pricing and underwriting strategies.
- Lack of Customization: Traditional risk assessment models often fall short in customization, primarily relying on broad population characteristics and generic disease spread models. Premiums are typically determined based on claims rates from specific age groups, neglecting the unique risk factors of individual policyholders. This one-size-fits-all approach can lead to pricing inaccuracies, leaving insurers vulnerable to excessive risk or making coverage unaffordable for certain demographics.
- Challenges in Adapting to Evolving Risks: Most of the underwriting models are based on traditional measurements of risk and struggle to keep pace with the rapidly emerging diseases and climate change-related natural disasters. As new threats arise, the inability to swiftly adapt can hinder effective risk management and leave organizations exposed to excessing claims reimbursements.
- Lack of Data Integration: Though health insurance companies have a good handle on the inpatient hospital data, they don’t good a good job in integrating this data with patient generated health data from fitness trackers or other data-enabled devices. This results in the insurer looking at the population only from a clinical perspective and not considering a holistic approach to collecting patient centric data. This results in creating an underwriting model that is biased by considering only a certain segment of a population.
The Critical Role of Data in Underwriting
Data Preprocessing
Data serves as a bedrock for accurate risk assessment and policy pricing. As the pace of data collection accelerates, insurance industry grapples with processing a huge number of applications submitting both structured and unstructured data, residing in the form of texts, images, PDFs, emails, videos, and forms. Extracting insights from these massive datasets is often tedious and time-consuming.
Since real-world data is inherently siloed, transactional and not standardized, it may not be able to paint a complete story of the underlying population or be able to mine one-off member specific insights. It is necessary that we transform this real-world data into a suitable format so that we can uncover strategic long-term trends as well as detailed member specific data at a granular level. It involves several steps including data cleaning, integration, enrichment, and transformation. This cleaned and transformed data then can play a pivotal role in automating underwriting workflows and analytical processes, which can drastically reduce the policy issuance time.
Data Democratization
Conventionally, data access within insurance companies has often been restricted to specific departments like underwriting or actuarial teams. To unlock the power of the data thus collected and integrated so far, a fundamental shift towards data democratization is needed at an organizational level. This means making data readily accessible and understandable across different teams, have a standard data definition and allowing a broader range of individuals to contribute data. This diversity not only enriches the data model but also mitigates biases that can arise from overly relying on any single parameter.
While doing this, it is important to have a robust governance framework with clear guidelines on data stewardship, quality, access controls and security to ensure responsible data utilization across the organization. Also, providing self service BI capabilities through low code no code BI platforms like Power BI, can greatly enhance the data accessibility outside the core IT organisation and encourage non-technical users as well to build custom reports and dashboards that can help them to make data driven decisions in their core business workflows.
The Role of AI in Underwriting and Risk Assessment
AI enhances data-driven decision-making by automating the analysis of vast datasets, enabling insurers to extract insights more efficiently and accurately. By leveraging machine learning and natural language processing, AI can identify patterns and correlations within data that may not be immediately apparent to human analysts. This advanced capability facilitates more precise risk assessments and enables personalized policy pricing, ultimately resulting in improved customer satisfaction.
Real-world Use cases of AI-Driven Risk Assessment
With the advent of AI technologies like generative AI and Large Language Models (LLMs), it is now possible to combine enterprise data with associated public domain data and come up with a comprehensive risk assessment, which can help an underwriter to price the premium at an individual level, by taking into consideration various scenarios that were till now not possible.
Let’s explore some AI-driven risk assessment with real-world examples.
Personalized Risk Profiling
Quick and accurate identification of client-specific risks helps underwriters to reduce the policy issuance time from days to minutes.
Consider the scenario:
AI can analyze Robert’s running data from fitness apps like Strava or Apple Health (with his consent) to gain valuable insights into his fitness level and potential health risks. These additional insights can be combined with his personal health records data (weight, blood sugar levels, and blood pressure) to provide a granular understanding of his risk profile.
The underwriter can also analyze Robert’s medical history, lifestyle habits (smoking and alcohol consumption), credit scores, hobbies (extreme sports participation) and social media sentiments which is often overlooked in a traditional risk assessment.
These additional data points empower the underwriters to make precise risk assessments, predict the likelihood of future claims and make well informed underwriting decisions with unprecedented speed and accuracy, ensuring a fair premium for Robert, based on his health-conscious lifestyle.
Insurance Price Optimization: Integration of Real-Time Telematics Data
Traditional actuaries rely on static and historical data that don’t reflect individual risk profiles. AI provides intelligent suggestions on dynamic insurance pricing by calculating optimal premiums based on a comprehensive analysis of individual risk profiles.
Consider the given two scenarios:
Personalized Premiums with device telemetry data: The integration of real-time device telemetry data enables underwriters to analyse David’s driving data that can reveal patterns about his driving style (smooth braking and adherence to speed limits), mileage, type of roads he frequents (highways or city streets), vehicle service history, location and time of use (avoids late-night commutes), etc. to further refine his risk profile. As a result, AI can provide David with a lower premium that accurately reflects his responsible driving behaviour.
Usage-Based Premiums: Similarly, Jane can choose a pay-as-you-go insurance plan based on the actual mileage she drives. AI can analyse Jane’s telematics data, providing real-time feedback on her driving habits and allowing for continuous risk assessment and potential premium adjustments, if her driving patterns change.
Generative AI-Powered Underwriting Assistant: Enhanced Customer Service
The advent of Generative AI and LLMs opens a new horizon for insurance companies to deliver exceptional customer experiences, increase efficiency, and build stronger customer relationships. The integration of conversational AI chatbots and virtual assistants helps insurers facilitate round-the-clock support for policyholders, including answering questions, clarifying policy details, offering personalized policy recommendations, and even guiding them through claim submissions, fostering customer trust, satisfaction, and increased retention rates.
For instance, after a minor car accident, Mark needs to file a claim.
Mark can initiate the claims process with a GenAI-powered chatbot that can handle tasks such as verifying policy details, requesting incident documents, submitting relevant details and even provide updates on his claim status, without having to talk to a customer support agent. Generative AI enables personalized customer interactions, avoiding back-and-forth communication with agents, thus reducing wait times and ultimately leading to a higher customer satisfaction.
How iLink Digital can help you?
As we can see from the above use cases, the benefits of AI-driven risk assessment are immense, and it can improve the overall operational and financial efficiency of the insurer. iLink Digital is a global IT services firm specialized in Data and analytics services, that can help insurers create a robust data management strategy and identify use cases that can help them to run their processes better. Some of our core services include:
- Data Integration: We help insurers to create a wholistic view of the member data by integrating data from both structured and unstructured sources.
- Data Governance: We help our insurers to establish a best-in-class data governance model that can help them to manage their data assets, mitigate security risks, and adhere to regulatory requirements, ultimately leading to improved trust and accountability.
- Data visualization: Our expertise in data visualization empowers underwriters to analyse complex data and derive actionable insights through business-friendly reports and dashboards. These reporting solutions can be used by different business units to better understand the business and identify opportunities for growth.
- Generative AI Workflows: With our deep expertise in Generative AI, we help underwriters streamline complex workflows, offer personalized recommendations, generate summaries, and expedite risk assessment, enabling quicker and more accurate decision-making.
Explore how iLink modernized the data infrastructure for a health insurance provider!
If you are looking to fine-tune your underwriting process or identify any AI-driven use cases, please Get in touch with our AI experts to embark on a transformative journey.
About Author
Saravanan Sadaiyappan, Head of Healthcare and Life Sciences at iLink Digital, is a seasoned leader with deep expertise in healthcare software and product development. He has a strong vision of building new business models by intersecting diverse disciplines through rapid prototyping, emphasizing agility in processes and technology to drive successful outcomes. Under his visionary leadership, iLink Digital remains at the forefront of technology advancements in the healthcare landscape.