Generative AI in Cybersecurity: A Double-Edge Sword or Cyber Shield?
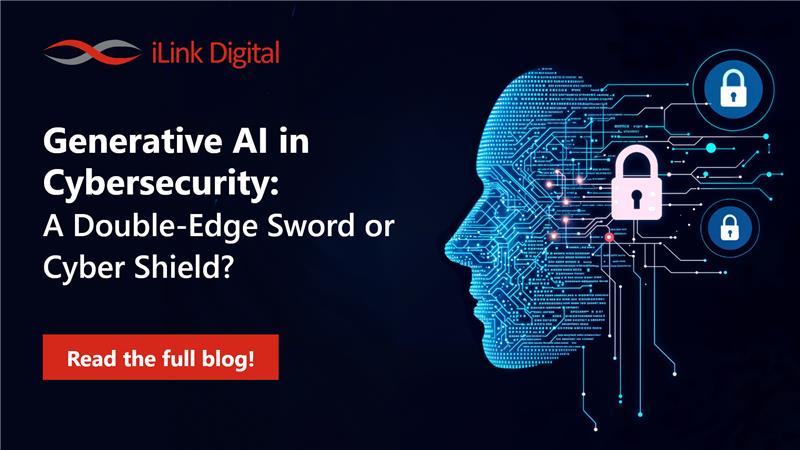
Introduction
According to Gartner, 80% of businesses are likely to adopt Generative AI in their digital footprint, yet 60% remain unprepared to manage the associated cybersecurity risks. It is no longer news that Generative AI is marking a transformative impact across industries; businesses are already deriving value and material benefits from GenAI adoption.
While GenAI revolutionizes creative processes and operational efficiencies, it is often characterized as a double-edged sword in cybersecurity. While LLMs empower defenders with advanced tools to identify and prevent complex threats, they also empower adversaries to find potential vulnerabilities in our infrastructure and network to launch highly targeted phishing and social engineering attacks, craft undetectable malware, and deploy deepfakes to deceive victims.
This dual nature of GenAI underpins the growing need for organizations to strike a balance leveraging Generative AI’s potential while mitigating its risks. Organizations must adopt ethical and responsible usage practices, implement robust security frameworks, and invest in AI-driven defense strategies to mitigate the evolving threat landscape effectively.
Generative AI in Cyber Offense: A Sword
As cybercrime continues to mature with AI technology, businesses and enterprises must also leverage AI to strengthen our security, protection, and response capabilities. The versatility of GenAI has introduced new attack surfaces, including prompts, responses, training data, RAG data, and models. This dynamic risk landscape also opens the doors for amplified risks, including data leakage, oversharing, prompt injections, hallucinations, and model vulnerabilities.
Generative AI assists adversaries in deploying highly targeted individuals by generating fake identity elements, including high-quality images, deepfakes, and voice impersonations. From identifying the targets to successfully executing the attacks, AI empowers adversaries by automating labor-intensive tasks, freeing them up to perform more nuanced tasks.
Here are the notable emerging threats on the block, posed by Generative AI:
Cross-Prompt Injection Attack (XPIA): This is a more sophisticated method also known as indirect prompt injection and represent one of the most concerning risks of LLM deployment. These attacks exploit vulnerabilities in how systems integrate third-party controlled inputs, such as email messages, website content, or documents, into AI-generated prompts.
Malicious Payload Insertion: Attackers exploit vulnerabilities by embedding malicious instructions within user-controlled data sources. For instance, a seemingly benign email attachment could cause an AI-driven email client to execute unauthorized commands. As these payloads can easily bypass conventional security guardrails, it can easily exfiltrate sensitive data or trigger malicious workflows without human intervention.
Credential Exploitation: XPIA can force LLMs into running tasks using the victim credentials, granting attackers unauthorized access to sensitive systems.
Data Exfiltration: These attacks can stealthily extract confidential information by manipulating AI outputs, leaving minimal trace for forensic investigation.
AI-driven phishing Emails: The dawn of LLMs can craft personalized phishing emails or messages to target individuals by analyzing their communication style, behavior, and preferences. A 2023 study showed AI-crafted phishing emails achieved a 78% success rate compared to 52% for human-written ones.
54% of phishing campaigns targeting consumers impersonated online software and service brands
AI-driven Social Engineering: AI models can mimic human behavior convincingly, tricking victims into sharing sensitive information. For instance, attackers leverage AI to create highly convincing LinkedIn profiles, impersonating recruiters and targeting executives for spear-phishing campaigns, resulting in security breaches at large scale.
Zero-Day Exploits & Vulnerability Discovery: As GenAI is capable of analyzing vast code bases, it can detect patterns indicating potential vulnerabilities, accelerate zero-day exploitation and discovery, and significantly reduce cybercriminals’ time-to-attack.
Bypassing Security Measures: AI models can be trained to mimic user behavior or generate inputs that can trick biometric security systems, CAPTCHAs, and other AI-based security solutions.
Disinformation and DeepFakes: GenAI can generate realistic fake media by using synthetic media, known as “deepfakes,” with the target of impersonating individuals by bypassing identity verification systems. A cybercriminal may use shallow fake mail and text messages to convince employees to authorize their fraudulent transactions. As we look forward to 2025, these sophisticated attacks will cause major challenges, especially in identity verification.
According to Microsoft Defense Report 2024, Biometric spoofing and the creation of synthetic identities using GenAI have become more prevalent in e-commerce payment fraud. AI-generated deepfakes can bypass even robust bypass biometric security measures in many payment transactions. Additionally, fraudsters use AI to craft realistic synthetic identities to manipulate merchant customer support functions.
According to Gartner, “BY 2026, 30% of enterprises will not consider biometric identity verification and authentication as a reliable solution.
As deepfakes become more prevalent in the digital business landscape, organizations must leverage robust countermeasures, such as requiring additional verification for payment transactions.
Generative AI in Cyber Defense: The Shield
The Rise of Security Copilots: A New Arm for Defenders
The rise of Security Copilots amplifies defender’s efforts by optimizing resources and scaling cybersecurity endeavors with greater precision, scale, and speed. This is particularly crucial considering the significant shortage of skilled cybersecurity workers, which poses one of the biggest challenges in the field of cybersecurity. This groundbreaking innovation amplifies defenders’ efforts by optimizing resources and scaling cybersecurity endeavors. It is really a shortage of skilled SOC analysts, regulatory compliance demands, and ever-evolving sophisticated adversaries.
- On average, it takes 277 days to identify and contain a breach, with 207 days for detection and 70 days for containment.
- According to a 2023 study, Microsoft Security Copilot equipped novice users to achieve 26% faster performance with 44% greater accuracy across all security tasks.
The advent of Generative AI holds incredible potential for introducing new defense strategies and methods. From earlier threat detection to prompt triaging and incident response, Generative AI has marked a significant leap forward in the dynamic threat landscape. For instance, it enables persistent systems that constantly monitor for vulnerabilities and promptly address any breaches.
Enhancing Threat Detection and Response:
Generative AI excels at analyzing massive datasets, enabling real-time detection of anomalies and patterns that indicate cyber threats. AI-driven systems like Microsoft’s Sentinel can identify malware variants, detect subtle changes in network traffic, and suggest countermeasures.
Incident Response and Threat Containment
LLMs are proving indispensable in speeding up the triage process by analyzing patterns from previous incidents and applying relevant policies. LLMs can recommend immediate response actions with high precision. Moreover, it can automate incident containment, such as isolating affected systems to prevent malware spread. Security Orchestration, Automation, and Response (SOAR) platforms powered by GenAI streamline workflows and reduce response times significantly.
Real-World Example: How LLMs saves 20 hours per person in Triaging Requests and Tickets
At Microsoft, an internal response team is overwhelmed with large requests and tickets, an average of 25 security requests per week. This volume is projected to double within six months. Traditionally, the initial triage for a single request took approximately three hours, consuming significant resources and slowing response times. LLMs significantly reduce this time to mere seconds by leveraging historical data, organizational policies, and insights from similar cases, ultimately saving at least 20 hours per person per week and enhancing the productivity of defenders.
Moreover, Generative AI platforms excel at automating repetitive and time-consuming tasks such as patch management, log analysis, and playbook execution, enabling security professionals to focus on high-priority incidents.
Best Practices and Recommendations in Generative AI Risk Management
As the rapid adoption of generative AI accelerates, addressing its accompanying threats is inevitable to fully harness its potential. Organizations should prioritize a proactive approach to securing AI systems.
Here are a few actionable recommendations for navigating the Generative AI risk landscape
- Establishing Clear Policies and Guidelines: Define ethical usage policies and train teams to align with compliance requirements.
- Risk-Based Containment Strategies: Apply risk-based containment strategies using tiered product access and customer behavior monitoring to manage the malicious use of AI and fake identities.
- AI-powered Guardrails: Integrating AI-specific safeguards, such as prompt-level encryption or role-based restrictions.
- Enhanced Collaboration: Fostering collaboration between developers, stakeholders, policymakers, and end-users to create robust defense frameworks by regularly gathering information about adversaries’ tactics.
- Conducting Regular Risk Assessments: Perform AI-specific threat assessments to identify potential vulnerabilities in AI-driven systems through threat modeling.
- Implementing Robust Data Governance: Ensure sensitive data is not inadvertently used in AI training models, reducing the risk of data breaches.
- Promoting Awareness and Education: Equip teams with knowledge about GenAI’s potential threats and defensive applications.
- Enhancing Transparency: Regularly audit AI models to understand their decision-making processes and ensure they align with security objectives.
The Way Forward:
Cybersecurity stands at the forefront of global concerns; Generative AI in cybersecurity opens new avenues for both progress and perils. Being a Microsoft Gold Level partner, iLink well-positioned itself as an “AI First” company, offering a proactive and balanced approach, combining advanced AI defense mechanisms with robust governance frameworks and cross-sector collaboration to navigate the evolving threat landscape. We’re deeply committed to prioritizing security and responsible AI implementation, ensuring the safety and integrity of our advanced systems.